Contact us and see what NetOwl can do for you!
Reduce Customer Churn with Sentiment Analysis
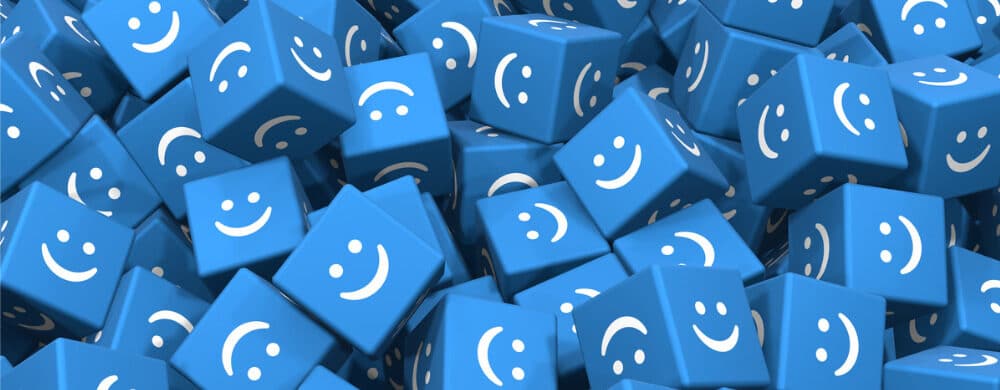
It’s Imperative for a Company to Reduce Customer Churn
Customer churn is a very important metric for a business. It refers to the number of customers who cut ties with a company during a certain period. There are multiple ways of calculating churn, but a basic one is simply to divide the number of customers you lost during the last quarter by the number of customers you had at the beginning of the quarter. For example, if a company had 100 customers at the beginning of the quarter and lost four of them during the quarter, then its churn rate is 4/100 or 4%. Minimizing churn is extremely important, as it’s much more economical to retain customers than to acquire new ones.
Companies need to understand what is motivating customers to leave (and also to stay). The traditional way is to perform analysis on both lapsed customers and those who have stayed, e.g., by doing surveys, and to compare the two sets. This is fine as far as it goes, but it’s a bit limiting: Surveys are always going to reflect a fairly narrow group of your customers, as it will consist of individuals willing to fill out a longish questionnaire. And fundamentally, responses to questions will also be biased by the questions asked, so you might be missing out on critical information just because you didn’t ask the right question.
Using Sentiment Analysis to Understand Churn
A better approach, and one which has become possible due to advanced technology, is to analyze all the unstructured text comments that customers leave in the many channels that they use to communicate with a company, particularly at its web site and on social media. Customers leave multitudes of unstructured text comments at different places on the site (the ordering page, the Contact Us page, etc.) and on social media. This is very rich and informative data, which is tedious and hard to analyze manually but would give a broader and in-depth view of what’s working to keep customers and, alternatively, what is driving some away.
However, advanced text analytic technology – Sentiment Analysis – can provide such a holistic view of the customer experience.
How Sentiment Analysis Works
Sentiment Analysis aims to understand the emotional attitudes of customers. The first generation of Sentiment Analysis technology simply looked for emotive keywords such as “wonderful” or “terrible.” However, this approach couldn’t figure out exactly which aspects of, say, a product or service the sentiment was targeted at.
More advanced versions of Sentiment Analysis use AI to analyze the linguistic context and derive a much more fine-grained analysis of the sentiment. This is known as Entity-Based Sentiment Analysis and provides a much more detailed analysis.
How Entity-Based Sentiment Analysis Can Be Used to Analyze Customer Comments
Here’s a typical customer feedback comment that might be left on a social media:
“The X product is pretty good, but its customer support is soooo slow.”
There are two sentiments being expressed here, one positive and one negative. The output of a Sentiment Analysis product would look like this:
-
- sentiment: positive
- sentiment predicate: good
- entity: X product
-
- sentiment: negative
- sentiment predicate: slow
- entity: customer support
The two unstructured sentiments expressed by the customer have been transformed into structured data which can then be quantified and aggregated over a very large set of such comments. Sentiments regarding all aspects of a company and its products or services can be tracked. One popular way of displaying such structured data is via a dashboard, which allows tracking of sentiments over time. This gives a good view of areas where a company is improving its performance versus the reverse and can also alert a company to emerging issues.
Furthermore, by analyzing sentiment for competitors using publicly available data on social media, companies can assess their market strengths and weaknesses relative to their competitors. For instance, competitor A may be praised for more economical or faster shipping, which may represent a risk of losing one’s customers. Competitor B may be dinged for low quality, which may be an opportunity to highlight the quality of one’s products and services to attract those dissatisfied customers.
In sum, Sentiment Analysis makes it possible for companies to understand precisely what is keeping customers happy and what is potentially driving them away.