Contact us and see what NetOwl can do for you!
How Sentiment Analysis Can Help Election Campaigns
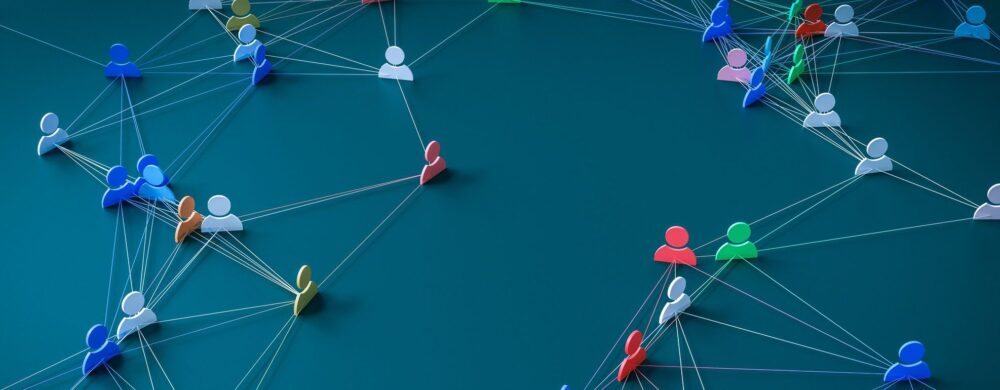
Sentiment Analysis Is an Advanced AI technology that Gives Political Campaigns Unprecedented Insight into Public Opinion
Sentiment Analysis, an AI technology, has become a critical tool for political campaigns to analyze public opinion. It enables campaigns to devise more effective strategies for attracting voters.
Particularly with the growth in popularity of social media platforms, a massive amount of unstructured text data is produced every day by millions of users. Using Sentiment Analysis, campaigns can analyze this data in order to understand public attitudes towards various issues and politicians. They can see which issues are most salient in people’s minds and what attracts them ‒ or doesn’t attract them ‒ to a candidate. Sentiment Analysis operates at scale and gives campaigns the capability to monitor in real-time what the public thinks of them.
Sentiment Analysis Offers Many Specific Benefits to Political Campaigns
- Campaigns can identify areas of strength and weakness as reflected in public opinion and can enable them to devise effective counter-messaging. For example, a campaign can identify tweets going viral that contain derogatory claims about its candidate and quickly issue its own counter tweets. Here’s a typical negative tweet:
“I hate Terry Dunn’s urban development plan. It’ll increase traffic and noise in our downtowns.”
- Campaigns can assess the public response to specific ads and can then tweak those accordingly. Going beyond simple likes and emojis, campaigns can analyze the natural language comments about their ads and see if they are trending positive or negative:
“I saw the new campaign ad for that moron Terry Dunn and I’m fed up with his personal attacks.”
- Campaigns can better understand the issues that are important to specific groups or localities and can then fine-tune their messaging for those groups:
“Politicians in DC have forgotten about our communities here in Detroit. We need jobs!”
- Campaigns can identify and track the competing campaigns and candidates. They can analyze the public response to the opposition’s ads. They can study the attitudes to the opposition’s candidate or to the issues it is emphasizing. This will allow a campaign to gain a good understanding of the opposition’s strengths and weaknesses and help to develop effective attacks:
“Susan Todd has a better track record in economic policy.”
How Sentiment Analysis Works
Using AI techniques, Sentiment Analysis identifies people’s attitudes in unstructured text data such as that found in tweets and other social media sources.
Basic versions of Sentiment Analysis as offered by some vendors can identify positive or negative sentiments in a text or a sentence by looking for, in a simplistic fashion, sentiment-bearing words such as “great” or “terrible.” They do not, however, specify what the sentiment is targeting because they do not understand the context in which those words occur. It cannot, for example, process a text such as the following:
“Susan Todd’s latest campaign ad is awful, but her speech last night was pretty good.”
Here two sentiments are being expressed – a negative one towards the campaign ad and a positive one towards the speech. What is needed is a technology that can recognize that two sentiments are being expressed and can associate them accurately with the politician’s ad and speech respectively.
The more advanced version of Sentiment Analysis, Entity-Based Sentiment Analysis, achieves these goals. It is able to identify not only sentiments but also the target of those sentiments and any associated entities.
Advanced Entity-Based Sentiment Analysis also makes possible the aggregation of sentiment found in social media posts. This is because it structures all the sentiments and entities it identifies. The following examples show typical outputs of Advanced Entity-Based Sentiment Analysis:
- “Terry Dunn’s recent campaign ad is really terrible.”
- Sentiment Type: Negative
- Sentiment predicate: terrible
- Target: campaign ad
- Associated entity: Terry Dunn
- “David Johnson is the most repulsive politician I’ve ever seen.”
- Sentiment Type: Negative
- Sentiment predicate: most repulsive politician
- Target: David Johnson
- “I can’t believe how expensive my groceries are nowadays.”
- Sentiment Type: Negative
- Sentiment predicate: expensive
- Target: groceries
- “I’m optimistic about Terry Dunn’s health care proposals.”
- Sentiment Type: Positive
- Sentiment predicate: optimistic
- Target: health care proposals
- Associated entity: Terry Dunn
- “Terry Dunn’s social security policy is just what we need.”
- Sentiment Type: Positive
- Sentiment predicate: need
- Target: social security policy
- Associated entity: Terry Dunn
In these examples, the sentiment type, Positive or Negative, is always identified. In addition, the component elements of the sentiment ‒ the sentiment-bearing words, the target, and the associated entity ‒ are specified.
This structuring allows sentiments to be aggregated, giving campaigns a statistically grounded view of what the public thinks about them. The time stamps associated with the social media posts can also give campaigns a view of how public attitudes develop over time. This supports a dashboard-style display and supplies specific quantitative evidence of how public sentiment is trending.
Summary
Sentiment Analysis is an effective AI technology for monitoring public opinion in the context of a political campaign. It gives the candidates and their staffs real-time access to what the public is thinking about the candidates’ personal qualities, their stances on the issues, how good their messaging is, what views the public has of their opponents, and many other aspects of a campaign.