Contact us and see what NetOwl can do for you!
How Name Matching Helps Money Transmitters with CRM
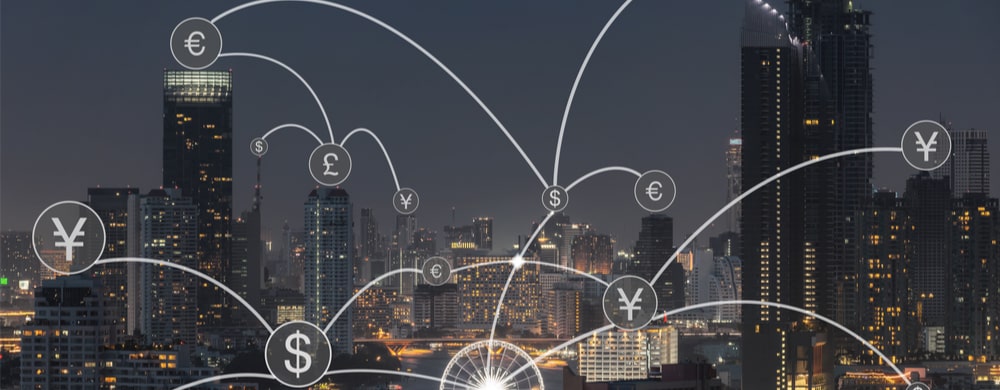
With increased emigration world-wide, international money transfers between individuals (“remittances”) have become a lucrative business. The World Bank estimated the volume of remittances in 2018 to be US$689 billion, of which $528 billion is going to developing countries. Banks and money transfer companies like Western Union are the traditional means of transferring funds internationally, but increasingly money transfer companies are moving into the niche with a much more economical business model suitable for clients who don’t deal with banks.
As they grow in size and number, money transfer companies need to conduct what financial institutions such as banks have traditionally done: Know Your Customer (KYC) and AML compliance, fraud detection, and Customer Relationship Management (CRM). The last is particularly important, as money transfer companies need to understand their customers, like any other business, by establishing a unified database of their customer data. A major motivation in doing this is to determine the total picture of transfers and any other purchased services and products for each customer.
How Name Matching Can Enable CRM for Money Transfer Companies
An exciting new technology, Name Matching, has emerged that will allow money transfer companies to develop an effective CRM strategy.
A major difficulty in establishing a unified database for customers is that their name may vary from one transfer to another. Some causes of this include the following:
- Word order variations (Augusto Jimenez – Jimenez, Augusto)
- Initials (Juan Gonzalez – J. Gonzalez)
- Abbreviations (Avenida – Av./Avda.)
- Nicknames (Eduardo – Lalo)
All of these may, of course, be accidental or intentional.
The records can also be in English or other languages and language scripts, resulting in differences in records due to transliteration variations (e.g., Haydar bin Battuta el-Sisi – Haydar bin Battutah al-Sisi). The record fields may also contain data other than names: dates, addresses, and a variety of numeric values, all of which need to be accurately matched.
Modern matching techniques have to be robust in the face of all these potential record discrepancies, not only individually, but in combination. They must also scale to meet today’s Big Data-sized challenges in order to support real-time screening of very large record sets.
How Name Matching Meets the Challenge
Name matching is a critical technology to support the requirements of money transfer companies. Here are some crucial features of an effective and accurate name matching tool:
- High accuracy. Effective name matching requires that it be highly accurate, minimizing false positives (not making bad matches) and false negatives (not missing good matches). Given the wide range of ethnic name types, name matching must also be able to handle the differing characteristics of names from all over the world.
- Scalable and real-time. Name matching must be engineered to enable high-volume, real-time matching of prospective customer records.
- Tunable. A name matching product must allow the user to create business rules to determine what combination of record attributes should be matched and how important each attribute is to the overall matching score.
In sum, money transfer companies need name matching in order to achieve real value-producing CRM.