Contact us and see what NetOwl can do for you!
How Entity Extraction Identifies Adverse Information on PEPs
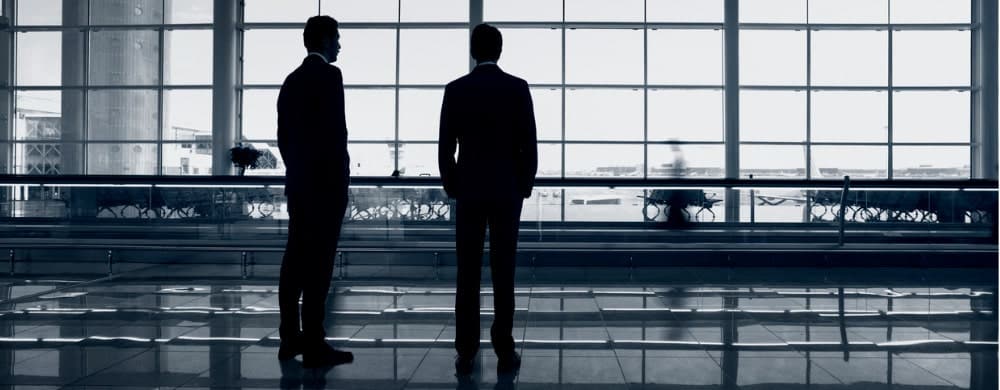
In today’s global economy, financial institutions like banks and insurance companies are exposed to new types of risk. One such type of risk is known as Politically Exposed Persons (PEP). PEPs are those individuals who have gained a prominent public position in a foreign country. Financial institutions, which frequently deal with PEPs, must avoid doing business with any such individual that has been involved in corrupt or criminal activities or else they risk liability and reputational loss. It is therefore critical that a financial institution be able to check any adverse information about such people.
Data providers have emerged that offer this information regarding PEPs. They offer services that aggregate, by way of a unified search, an enormous amount of material. Standard PEP sources include the CIA World Leaders List, World Statesman, the European Parliament Directory, and many, many others. The data providers typically offer a SaaS search capability where their subscribers performing due diligence can search on PEPs.
The official sources listed above are an excellent resource, but to be exhaustive and up-to-date they must collect daily information from news media and other sources such as social media. The challenge is that these data sources involve large amounts of unstructured data that is much too voluminous for manual review by humans. Think millions of news articles and social media posts per day and thousands of news sources from all over the world.
Here is where an AI-based technology called Entity Extraction makes it possible to capture data about PEPs from unstructured data in a scalable, real-time manner. Entity Extraction enables automated identification of semantic concepts in text such as named entities (“John Smith”). Advanced Entity Extraction goes beyond just named entities and identifies more complex semantic concepts such as relationships and events. Event extraction is particularly valuable to collect adverse information about PEPs because it identifies and analyzes events in texts such as any arrests, indictments, and crimes that a PEP has been involved in, in addition to information about other participants and the event’s date and time.
Relationship extraction is also critical to assess PEP risk because it makes it possible to discover who is associated with a PEP, including relatives and business associates. For instance, a financial institution would want to know if a PEP’s close relative (e.g., a spouse) or associate has been indicted for corruption. Entity Extraction analyzes the text and identifies phrases such as “X is the partner of Y.” By establishing the relationship between X and Y, any adverse information regarding X is available in a search for Y.
In sum, sophisticated PEP data providers are now looking to go beyond entity extraction and improve the precision (i.e., reduce false positives) of their current process by utilizing AI-based adverse event and relationship extraction capabilities.