Contact us and see what NetOwl can do for you!
Entity Extraction Is Critical for the Redaction of Documents
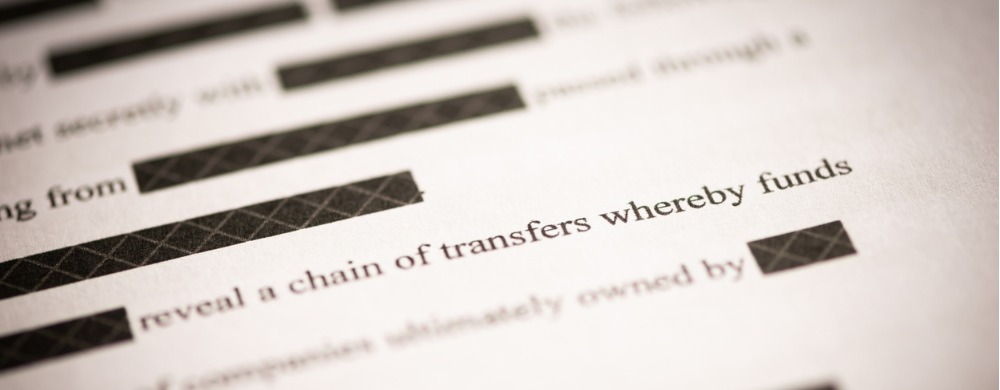
Redaction Is Necessary to Protect Sensitive Information
Redaction (also known as data masking) is a process applied to documents in order to obscure any sensitive information that shouldn’t be revealed to readers. For example, the prosecutor in the 2020 election subversion case recently released documents concerning the case that did not contain the complete text: they had multiple redactions. Typically in a legal prosecution, redactions are made to conceal any information that could:
- Compromise the investigation
- Reveal grand jury material
- Disclose law enforcement sources and methods
- Compromise the personal privacy of individuals mentioned in documents.
In addition, during a discovery process large numbers of sometimes very lengthy documents have to be redacted.
Traditionally, officials have just blacked out with a pen or a magic marker sensitive portions of a document. Of late, specialized software tools for redaction have come on the market.
Manual Redaction Is Slow, Costly, and Boring
Everyone’s aware of redaction failures that occur, for example, when the redacted material can be simply cut and pasted to a different application to make it readable. A more fundamental problem with manual redaction is that it is slow, costly, and boring. In complex discovery processes, for example, hundreds or thousands of documents have to be redacted. Searching for sensitive information in a 400-page document using a manual redaction application is a process that will be extremely prone to error and take a long time. Expecting a human to catch every occurrence of, say, phone numbers is unrealistic.
Fortunately, there is a solution which greatly accelerates the redaction process and also ensures it is accurate and cost-effective: Entity Extraction (aka Named Entity Recognition or Named Entity Extraction).
How Entity Extraction Supports Accurate and Cost-Effective Redaction
Entity Extraction, an AI technology, automatically identifies key semantic concepts in unstructured text, such as names of people and organizations. It does this not by having a long list of names. Instead, it uses AI technologies and makes use of the textual context surrounding a name, looking for clues that indicate a name is present. Entity Extraction recognizes names dynamically – its most significant contribution is that it identifies names that have not been seen before.
Entity Extraction automatically identifies and marks up all entities in unstructured text at high speed with great accuracy. This allows a human redactor to focus more quickly on what might be sensitive material. Entities extracted include:
- Person names
- Organization names
- Location names
- Phone numbers
- Email addresses
- Physical addresses
- Various numeric expressions such as social security numbers, license plate numbers, bank account numbers, credit card numbers, DoB, etc.
Entity Extraction also links the full forms of names, which appear upon first mention in a document, to their shorter forms, which typically appear later, e.g., “John Donaldson” and “Donaldson” or “International Business Machines Corporation” and “IBM.” Entity Extraction is able to determine that “Donaldson” refers to the same individual as “John Donaldson,” and that if the latter needs to be redacted, so does the former. Furthermore, to keep the document coherent, short and long forms of names have to be replaced with a single stand-in label like PERSON1 or ORGANIZATION2. To accomplish this, it’s necessary to know which short and long forms refer to the same entity.
Here is an example of a partial (fictional) e-mail containing information in a legal case going through discovery:
“I spoke to Bob Hudson yesterday about the issue we’ve been worried about. Hudson told me that he had scrubbed the records thoroughly. This should help Acme Corporation and its subsidiary Buzz Corp. avoid any difficulties in the future. I’ll let Bill know that we’ve taken care of the problem.”
And here is a redacted version of the text. It is more readable than the kind of redaction where the sensitive information is just obscured, as the sensitive information has been semantically encoded by Entity Extraction:
“I spoke to PERSON1 yesterday about the issue we’ve been worried about. PERSON1 told me that he had scrubbed the records thoroughly. This should help ORGANIZATION1 and its subsidiary ORGANIZATION2 avoid any difficulties in the future. I’ll let PERSON2 know that we’ve taken care of the problem.”
Summary
In sum, then, Entity Extraction offers an effective way to reduce the time and expense associated with redaction. It enables faster and more accurate redaction of sensitive information by identifying and highlighting those elements which may need to be concealed. By semantically encoding what kind of entity is being mentioned, it makes the redacted text more readable. In this way, it dramatically reduces the time a human redactor needs to spend searching for the relevant elements.