Contact us and see what NetOwl can do for you!
Entity Extraction Helps Insurance Companies Model the Cost of Adverse Events
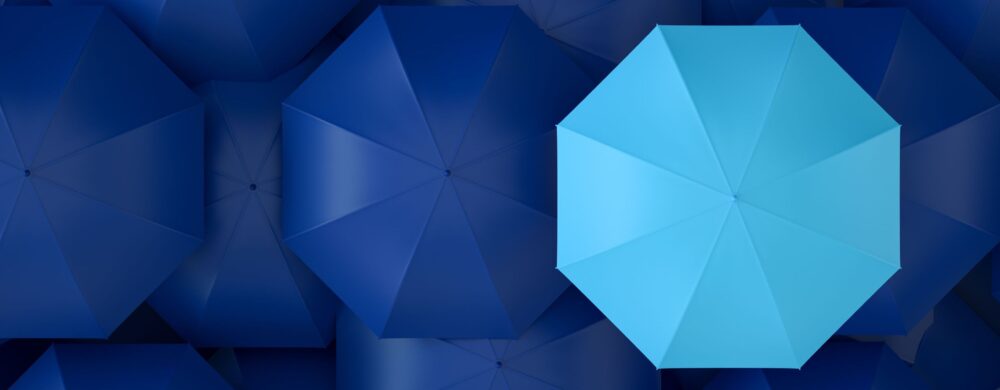
Insurance Companies Need to Accurately Predict the Cost of Future Adverse Events
Insurance companies need to understand the financial impacts of insuring losses due to any event that could cause havoc such as:
- Natural catastrophes (earthquakes, landslides, severe storms, wildfires, etc.)
- Human conflicts and crime (terrorism, unrest, violence, warfare, cyber data breaches, etc.)
Needless to say, climate change is a major contributing factor to the occurrence of many of these kinds of events, whether directly by causing widespread destruction or indirectly by causing famine and despair, which lead to unrest, mass migration, and violence.
Insurance underwriters must factor into their policies information about all of these events and the likelihood of their impacting an insurance customer. They need to reach the right balance between premium amounts and the risk of insured losses.
All kinds of insurance are affected by these factors:
- Homeowners
- Health
- Life
- Auto
- Business
- Identity Protection
- etc.
Modeling Future Adverse Events Require Data on Past and On-Going Adverse Events
As one example of the complex and multiple effects that affect the insurance industry, consider the on-going war in Gaza:
- At the time of the start of the war, the international insurance industry had several billion dollars of political violence and terrorism exposure in Israel, mainly in the area around Tel Aviv. Israeli people and organizations would purchase policies to protect their properties due to fear of Hamas rocket attacks. There has not been much damage in Israel so far due to the drop in these attacks subsequent to the Israeli incursion into Gaza, but the insurers may still have to consider raising premiums or cancelling policies if Hamas can launch more rockets in the future or if another party such as Hezbollah gets involved.
- Additionally, marine and aviation insurance to protect against damage inflicted due to the conflict will also likely see premium increases and maybe cancellation of existing policies and even withdrawal of insurance companies from the region if the conflict widens.
- There are also knock-on events: for example, holders of perishable goods stuck in a port due to ongoing hostilities also have insurance policies to compensate them for any losses.
Against this background of how much an insurer can be affected by a geopolitical event, it is imperative that it has a good understanding of the risks involved in insuring an individual or organization. It particularly needs to know as accurately as possible the maximum financial losses that could be inflicted by any adverse event.
Unstructured Data Contains a Large Amount of Information Relevant to Insurance Risk
A very large amount of data relevant to insurance risk is found in the unstructured data of the world’s various media – whether formal sources such as newspapers or less formal ones such as social media. In the past, insurance underwriters would have to manually and slowly compile the relevant information from these sources. In insuring a customer in a foreign country, it would assess, as one component of its research, the local geopolitical conditions from scanning the available sources on whether, for example, there was a significant threat of business disruption due to the activities of armed groups opposing the existing government.
Luckily, there’s an AI-based technology that can automatically collect information on such events: Entity Extraction, specifically an advanced form of Entity Extraction called Event Extraction.
AI-Based Entity Extraction Offers an Effective Way to Gather Information on Adverse Events from Unstructured Data
The key contribution of Entity Extraction is that it can process unstructured data at a very large scale and convert it into structured data that looks much like conventional database records. For example, take a look at the first sentence in a Reuters news article like the following:
DUBAI (Reuters) – Yemen’s Iran-backed Houthis said on Monday they launched attacks on Monday, May 27, on three merchant vessels in the Indian Ocean.
In processing this sentence, Entity Extraction uses AI techniques for analyzing syntax and semantics to identify:
- the nature of the event
- the participants
- the time and location of the event if given.
The output from Entity Extraction looks like this:
- Event Type: ATTACK
- Perpetrator: Yemen’s Iran-backed Houthis
- Target: three merchant vessels
- Place: Indian Ocean
- Time: Monday, May 27
(For more information on extracting events, see here.)
For the above example, Entity Extraction populates a pre-defined ontology consisting of items like Event Type, Perpetrator, Target, Place, and Time (among many other items). The values of those items are taken from the input text (“Yemen’s Iran-backed Houthis,” “three merchant vessels,” “Indian Ocean,” etc.). Some normalization of the values such as dates and locations may also be done.
Entity Extraction produces in this way a uniform, searchable/discoverable representation of the semantic content previously expressed in unpredictable, unstructured text data. Once stored in a database, an insurance underwriter can, for example, use an analytics tool or a dashboard to perform trend analysis and plot the attacks over time to see if they are showing a sizable increase or not.
The underwriter can also perform advanced semantic-based search against the extracted data, rather than conventional keyword search against millions of texts. For example, he/she can query for all ATTACK events in a certain PLACE at a certain TIME. The underwriter receives a structured and extracted data result listing all the ATTACK events with their PERPETRATOR, TARGET, PLACE, and TIME that meet his/her criteria and can drill down to the actual text for further inspection. This is a far more effective and efficient way to conduct research than combing through hundreds of texts returned by keyword search.
Summary
Advanced Entity Extraction offers an exciting new way that enables the insurance industry to gain a precise and comprehensive grasp of the risks potentially affecting the issuance of a new policy.