Contact us and see what NetOwl can do for you!
Entity Extraction and Redaction: Safeguarding Information Privacy
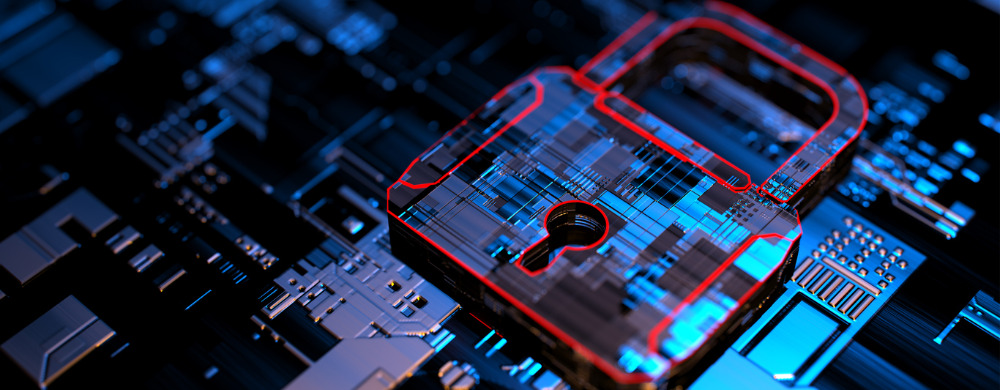
In today’s Digital Era, information privacy is a top concern for many individuals and organizations, both in the public and private sectors and across many industries, from health care to retail and banking.
There are numerous cases where organizations need to redact sensitive information or PII (Personally Identifiable Information) from their documents, most notably before sharing data with a third party. For instance, organizations involved in litigation must share data such as email messages as part of the e-Discovery process. Government agencies make incident reports such as vaccine adverse events available to the public for research and transparency. Police departments publish crime reports. Health organizations make health data available to researchers. While it’s usually straightforward to redact the “structured” part of these records/forms, the challenge often is that much of the same sensitive information is also present in free form notes and must be redacted as well.
It’s not just when sharing data with a third party that organizations need to redact sensitive information. It’s often also when sharing data within the organization itself. For instance, credit card numbers or social security numbers may need to be masked in call center records when they are shown to those that do not have a need to know that specific information.
Last but not least, in today’s environment in which data breaches are all too common, it is critical for organizations to protect themselves against liability and reputational loss by redacting PII from stored data whenever it is not required for any internal, regulatory, or legal purpose.
Redaction or Data Masking
Redaction, also known as data masking or identity masking, is about removing PII from unstructured data. There are multiple factors that contribute to making data masking a challenging task:
- PII spans a wide range of data types such as names of people, organizations, and locations, phone numbers, social security numbers, email addresses, mail addresses, dates of birth, license plates, credit card numbers, account numbers, etc. Some are fairly predictable in format and therefore easier to detect (e.g., dates of birth, credit card numbers). Others exhibit a large degree of variation and novelty. For instance, person names may be linguistically diverse (e.g., English, Spanish, Arabic, Slavic, Chinese), be highly ambiguous, and come in different word orders (e.g., first name + last name, family name + given name).
- It is not sufficient to redact full names. Short name mentions such as a last name or first name by itself must be redacted too.
- Some types of personal data may not be sensitive by themselves but in combination. For example, a person name by itself may not be considered sensitive but when combined with a date of birth or an address, it may become PII.
- To preserve the readability of the redacted documents, PII may need to be replaced with labels like PERSON1, PERSON2, CREDIT_CARD1, CREDIT_CARD2, etc., which requires keeping track of and numbering the various objects being discussed.
- The volumes of data to be redacted are often staggering.
It is easy to see how these factors make manually redacting PII a time consuming, costly, error-prone/ineffective, and simply impractical task, especially given Big Data volumes.
How does Entity Extraction Solve the Data Masking Problem?
Data masking is a perfect application for Entity Extraction technology in that Entity Extraction is specifically designed to detect concepts in text, primarily unstructured data like email, reports, doctor’s notes, etc.
NetOwl’s state-of-the-art AI-based Entity Extraction offers the following key capabilities to best address the redaction challenge:
- Broad semantic ontology. With over 100 types of entities out of the box, NetOwl offers a broad semantic ontology that goes beyond that of standard named entity extraction and covers the diversity of PII. Individual organizations can determine what set or combination of data types constitute PII and leverage the appropriate NetOwl output accordingly.
- Coreference resolution. NetOwl is not only able to identify full names and variants (often called ‘aliases’), but also resolve variants to full names through coreference resolution. Corerefence resolution allows for data masking that preserves readability. For instance, if coreference resolution determines that “John Campbell”, “John”, “J. Campbell”, and “Campbell” all refer to the same person, all 4 variants can be replaced with the same label (e.g., PERSON1).
- Robustness. Text data is often imperfect, especially if it consists of casual language like email or unedited text like quick call center notes. It may contain misspellings, inconsistent punctuation or capitalization, partial or ungrammatical sentences, etc. NetOwl has been trained extensively to be robust on a wide range of data sources, from well edited text (e.g., newspaper grade) to social media.
- Confidence scores. Depending on how sensitive the data is, a human in the loop may be required for the redaction process. NetOwl’s Entity Extraction outputs confidence scores so that a human reviewer can prioritize the decisions that the system was less confident about.
- Native document formats. NetOwl integrates document converters to handle hundreds of native document formats including popular proprietary formats like MS Word, and text-based PDF files.
- High throughput. NetOwl is engineered specifically for high-volume processing of multiple different data sources, which is critical in applications where time is of essence like e-Discovery.
- Easy integration. NetOwl integrates easily with databases, document and content management systems, portals, and other sources of electronic content. Its REST API supports easy integration into existing document processing workflows.
- On premise and in the cloud. For maximum flexibility, NetOwl’s Entity Extraction can be deployed both on premise or in the cloud for horizontal scalability, offering rapid processing of massive amounts of data.
To summarize, NetOwl’s broad entity ontology coupled with its coreference resolution, confidence scores, scalability, and robustness make it a state-of-the-art solution to the redaction challenge, thus safeguarding information privacy.